Discrepancies in Embracing Generative AI Technology
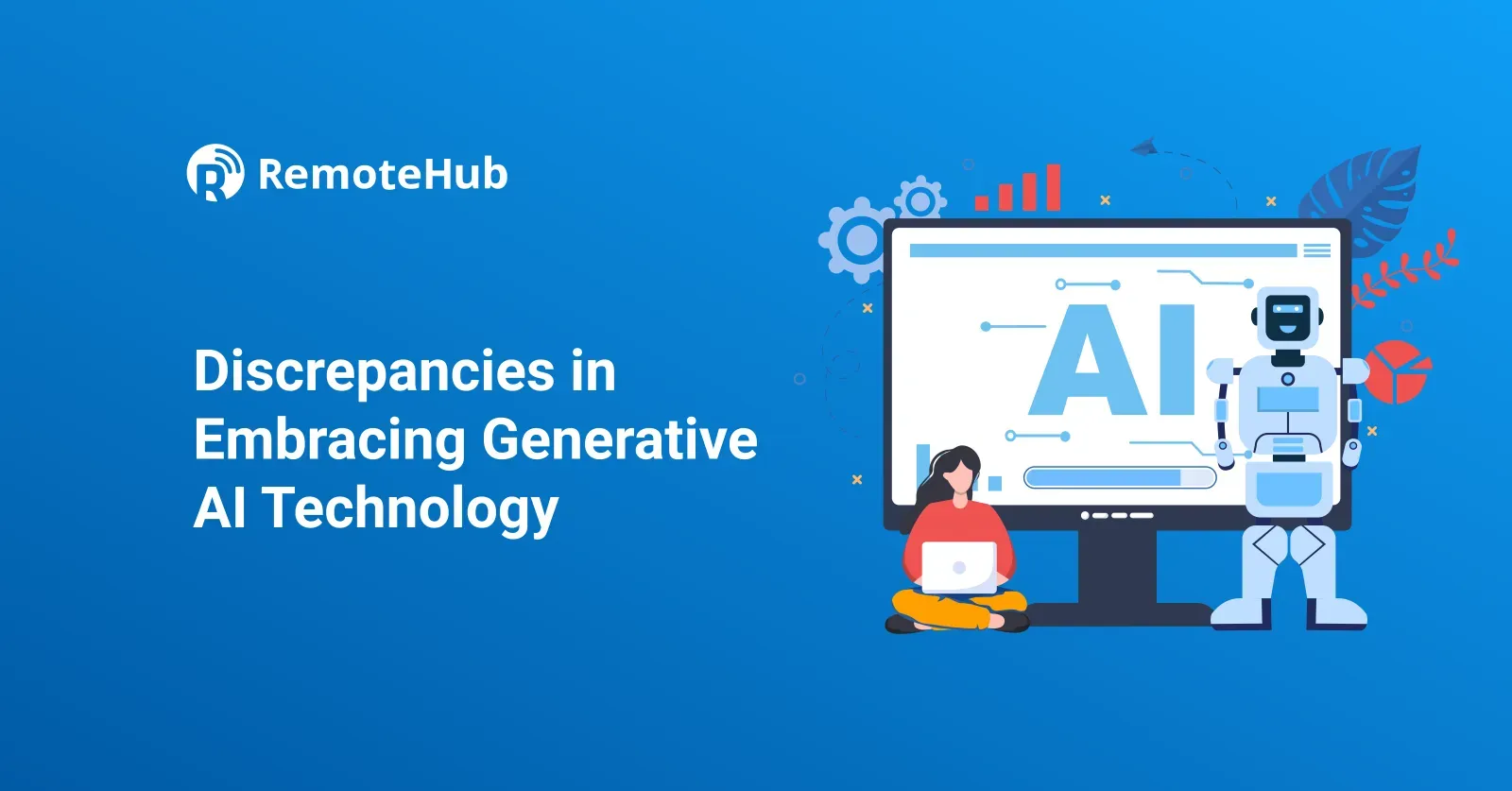
Generative AI refers to machine learning systems that can produce new content, designs, code and more rather than just analyzing existing data sets. Powerful models like DALL-E for images and GPT for text demonstration uncanny creative ability on par with humans.
Yet most enterprises hesitate fully embracing generative AI's potential transforming products and operations. Despite proven value creation applied properly, discrepancies hold many leaders back from wholehearted adoption.
This article will examine key contrasts preventing wider implementation across expectations, perceptions, measurable impact, and preparedness. We'll also construct an adoption framework addressing these gaps with best practices maximizing generative AI benefits while anticipating challenges. Soon these systems can transition from novel hype stirring concern to indispensable assistants fueling competitive advantage.
The Promise and Potential of Generative AI
Generative AI promises to radically expand human creativity, productivity, and innovation through tools mimicking imagination itself via impact across:
· New Product Conceptualization: Rapidly iterate visual designs, identify opportunities, and model options interacting directly with AI to externalize imagination into tangible prototypes and mockups.
· Personalized Content Creation: Generate customized graphics, product configurations, landing pages and proposals tailored to individual prospect needs and interest srather than one-size-fits-all static assets. Match messaging to what resonate sat scale through AI content expertise.
· Process Automation: From document generation to customer analysis, leverage AI to eliminate repetitive tasks plaguing enterprises allowing knowledge workers to focus on high judgement initiatives only humans can currently undertake based on experience and nuanced contextual interpretation. Leave rote mechanized work to automation.
· Enhanced Decision Making: Allow AI to rapidly process and construct massive data sets in to data stories with summarized analysis and recommendations for faster, more informed business decisions and forecasting especially effective in quantitative realms.
· Risk Assessment: Continually monitor anomalies and flagged incidents across operations using AI to catch issues human spot checks would likely miss while also running simulated incidents for crisis preparation by instantly generating thousands of scenarios identifying potential blind spots.
These capabilities make AI a rising star across nearly all industries yet puzzling hesitation slows adoption short of its full transformational potential. Let's examine why this discrepancy persists.
CONTRAST 1: Expected Impact vs Actual Implementation
Expectations: Leaders hear promises of revolutionary AI capabilities automating tasks, enhancing creativity, and optimizing decisions. The notion of generating novel ideas, designs and content instantly captures curiosity dreaming of results. Hopes run wild imagining game changing automation.
Reality: In practice generative AI proves narrower in current capabilities focusing largely on supplemental content augmentation rather than fully autonomous generation of entire creative works or complex analytical outputs. While great progress continues, hype exceeds actual unrealistic expectations. Leaders must embrace gradual adoption enhancing specific use cases rather than expecting sentient intelligence operating independently across enterprises overnight.
Mindset Shift
Generative AI indeed brings tremendous potential but remains narrow presently, best leveraged enhancing the work people already do rather than replacing jobs entirely in the short term. Set sights on targeted increments - maybe DALL-E improves designer's concept iterations 30% faster or GPT summarizations analyze survey response patterns 20% quicker than manual review. Define realistic expectations around beneficial but focused usage areas and metrics to deliver material uplift.
CONTRAST 2: Perceived Risk vs Existing Safeguards
Concerns: Despite productivity promises, brands worry embracing generative AI risks unintended harm - flawed medical diagnoses, offensive generated content, inventions better left undiscovered or automation eliminating jobs at scale. Caution slows adoption awaiting assurances models operate safely and ethically especially within influential fields like healthcare.
Truth: In practice, responsible generative AI prohibits harmful applications through extensive safeguards:
- Output Filtering using detection classifiers to screen undesirable or explicit content, misinformation, and biased language.
- Data Constraints limiting training inputs preventing ingestion of hazardous source material from inception.
- Model Sandboxes safely develop new algorithms isolated from real systems until deemed successful.
- Human-in-the-Loop Checks enable manual reviews flagging model errors before wide release.
- Bias Testing Suites proactively uncover skewed outputs or unfair performance prompting model fine tuning when necessary.
- Explain ability Frameworks make model logic transparent for audit preventing black box risks.
- Ethics Review Boards oversee development assessing risk/reward tradeoffs aligned to moral standards companies set adhering to "first do no harm principles".
Yes, risks exist, but responsible implementation minimizes downsides making AI assimilation safe using constraints and oversight.
Mindset Shift
Rather than write off generative AI as an inevitable liability, construct an ethical framework addressing risks upfront while allowing controlled usage improving key functions without unleashing unstable systems threatening brand reputations or public safety once adequate governance proves trustworthy model performance.
CONTRAST 3: Abstract Potential vs Tangible Examples
Challenge: Leaders struggle visualizing concrete applications of nascent generative AI capabilities. Vague hypotheticals confuse more than clarify practical integration opportunities tied to strategic priorities and existing pain points. Lofty visions fail catalyzing urgency to adopt among decision maker sand IT investors who control funding.
Reality: Enterprises often already sit on perfect generative AI use cases aligning to top organizational priorities solvable through these emerging tools, whether it's:
- Personalizing marketing engagement leveraging GPT’s natural language capabilities
- Having DALL-E rapidly mockup designer apparel concepts or graphics complementing new product launches
- Automating documenting contract summaries, prospect research synthesis and other administrative tasks bogging down departments
- Forecasting regional demand for supply chain insights by analyzing sales trajectories and external dataset shifts
Yet hidden in plain sight, these applications go unexplored, lacking examples demonstrating theoretical capabilities improving daily operations.
Mindset Shift
Rather than detached vendor pitches showing demos irrelevant to company needs, drive adoption by:
1. Starting use case ideation internally documenting known friction areas needing solutions
2. Researching cutting edge generative AI models potentially addressing these needs if applied.
3. Enlisting vendor partnerships running controlled pilots quantifying generative AI impact on priority business objectives over 6-12weeks.
Tangible pilots clarifying actual capabilities beat generic demos hypothesizing value. Quick wins spur investment confident in customer-centric examples vs gambling on vapid vendor promises alone.
CONTRAST 4: Current Costs vs Future Scalability
Pushback: Leaders balk at the hefty price tag contractual enterprise licenses command for privileged API access to leading generative AI models like GPT and DALL-E charging nearly $.02 per API call which quickly tallies requesting thousands of outputs like long form text generation. Short term costs appear disproportionate to opaque long term value.
Progress: Rapido pen-source innovation advances free and affordable alternatives to commercial models, reducing financial barriers to entry for wider experimentation. Options include:
- Anthropic's Claude - Promising rival offering cheaper bulk pricing training enterprise models internally rather than reliance on external APIs
- GitHub Co Pilot- AI coding assistant integrating predictions directly into developer workflows to accelerate software engineering leveraging crowdsourced learning
- Hugging Face - Open-source access to foundational transformer models like CLIP and BIG-bench questioning answering to build custom solutions
- Stable Diffusion - Impressive image generator rivaling DALL-E now completely free to run locally or host through services like Run Pod
Using these tools or sponsoring shared models distributed across industry groups drops costs from 5-6 figures to just hundreds monthly democratizing access from revenue limiting trials.
Mindset Shift
Rather than procure services pre-revenue or divert budgets from other priorities, harness increasing array of affordable open-sourced models commercially sponsored by partners invested already amortizing costs at scale. Cloud marketplaces also allow usage-based pricing only paying for actual generated outputs consumed. This portfolio approach reduces risk ensuring budget for success.
CONTRAST 5: Current Capabilities vs Future Potential
Pushback: Early results feel promising but limited compared to human capabilities causing some to underestimate long-term potential as AI algorithms evolve. Without foresight leaders lose patience for incremental gains on existing processes rather than exponential transformation achievable in coming years as databases and neural net works mature.
Reality: Rapidre search advances prove today's limitations quickly give way to surpassing human performance across more complex capabilities including:
- award-winning creative designs - AI not just assisting ideation but autonomously generating entirely novel yet appealing concepts beating industry veterans
- revolutionary scientific discoveries - like AI revealing new high-performance sustainable polymers and drugs matching years of human chemical PhD research
- hyper-personalized recommendations - anticipating individual nuanced interests better than any segmented marketing rules managed by analysts
- emotional intelligence and contextual reactions demonstrating reasoned decision-making capacities even in morally ambiguous situational scenarios confounding rule-based programming
And this just scratches the surface of documented groundbreaking applications in recent months!
Mindset Shift
Avoid shortsighted skepticism diminishing remarkable uptrends in problem solving prowess. Monitor exponential trajectory across metrics like accuracy, speed, use case scope and reasoning capacity as leading indicators of increasingly autonomous systems destined to transform business and society this decade through assistance AND automation alike.
An Adoption Framework for Generative AI
Beyond addressing perceived capability gaps inhibiting adoption, enterprise success necessitates a structured evaluation and implementation framework guiding assimilation centered on core pillars:
Tool Evaluation
Determine ideal models fitting business requirements across:
- Intended output type - Text, images, data etc.
- Use case complexity - Simple augmentation vs end to end automation
- Infrastructure requirements and costs - Cloud, on premise servers, local machine
- Customization flexibility - Can proprietary data/models be added to enhance reliability?
- Vendor support availability - Lacking in-house ML expertise assistance necessary
Pilot Scoping
Outline tightly scoped 10-week pilots assessing capability and impact including:
- Concise problem statement - Quantify pain points generative AI can help alleviate
- Success metrics - What observable improvement would warrant further investment?
- Participant criteria - Which internal teams participate providing context and evaluating outputs?
- Governance - What review mechanisms provide human checks flagging errors?
- Infrastructure - Where does the model run - cloud, local machines etc.?
- Cost analysis - Total trial budget allocation. Cost per generated output.
Trial Phase
Methodically test capability collecting data, feedback and examples across projected use cases while refining ideal configurations.
Weeks 1-2:Complete technical setup and run initial generations capturing baseline metrics.
Weeks 3-4: Share samples with pilot participants soliciting feedback applying improvements to the model.
Weeks 5-6: Analyze discrepancies between AI outputs and human subject matter expert recommendations documenting enhancement areas.
Weeks 7-8: Report interim metrics showcasing successes and construct remaining test scenarios for model.
Weeks 9-10: Complete final assessment deliverable quantifying generative AI impact and next phase scale recommendations.
This adoption roadmap bridges today’s uncertainty navigating cutting edge generative tools by constructing iterative feedback loops proving value safely outweighs risks.
Key Takeaways
Generative AI adoption lags ambitions despite incredible progress in recent years developing capabilities rivalling human creatives. Discrepancies holding many leaders back include:
1. Unrealistic expectations imagining autonomous systems over enhancing existing workflows presently.
2. Exaggerated risk perceptions downplaying extensive model safeguards ensuring responsible usage.
3. Unclear business applications struggling to tie academic hype to preoperational pain points generative AI can address.
4. High costs of leveraging external proprietary models are still maturing compared to open-source options emerging.
5. Shortsighted assessments underestimating exponential self-improvement trajectory towards surpassing limited human abilities this decade.
Construct an adoption framework focused on structured capability analysis, measurable pilot scoping and iterative feedback channels. This strategy bridges gaps embracing generative AI value immediately while anticipating even greater advances redefining entire industries long term through AI identified new opportunities and innovations. remotehub
Start small, think big!